京东用户购买意向预测
项目概述
京东作为中国最大的自营式电商,在保持高速发展的同时,沉淀了数亿的忠实用户,积累了海量的真实数据。如何从历史数据中找出规律,去预测用户未来的购买需求,让最合适的商品遇见最需要的人,是大数据应用在精准营销中的关键问题,也是所有电商平台在做智能化升级时所需要的核心技术。 以京东商城真实的用户、商品和行为数据(脱敏后)为基础,通过数据挖掘的技术和机器学习的算法,构建用户购买商品的预测模型,输出高潜用户和目标商品的匹配结果,为精准营销提供高质量的目标群体。
算法原理
1. Boosted trees
Boosted trees是一种集成方法,Boosting算法是一种加法模型(additive training),定义如下:
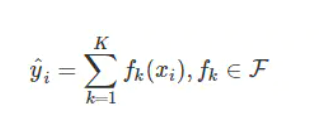

q(x)表示将样本x分到了某个叶子节点上,w是叶子节点的分数(leaf score)
下面通过一个具体的例子来说明:预测一个人是否喜欢电脑游戏,下图表明小男孩更喜欢打游戏。
2. 目标函数正则化
XGBoost使用的目标函数如下:
我们可以看出XGBoost在GBDT的误差函数基础上加入了L1和L2正则项,其中Loss函数可以是平方损失或逻辑损失,T代表叶子节点数,w代表叶子节点的分数。加入正则项的好处是防止过拟合,这个好处是由两方面体现的:一是预剪枝,因为正则项中有限定叶子节点数;二是正则项里leaf scroe的L2模平方的系数,对leaf scroe做了平滑。
接下来我们对目标函数进行目标函数的求解:

该目标函数表示:第i样本的第t次迭代误差函数,后面的推导基于上式。这种学习方式已经从函数空间转到了函数空间:
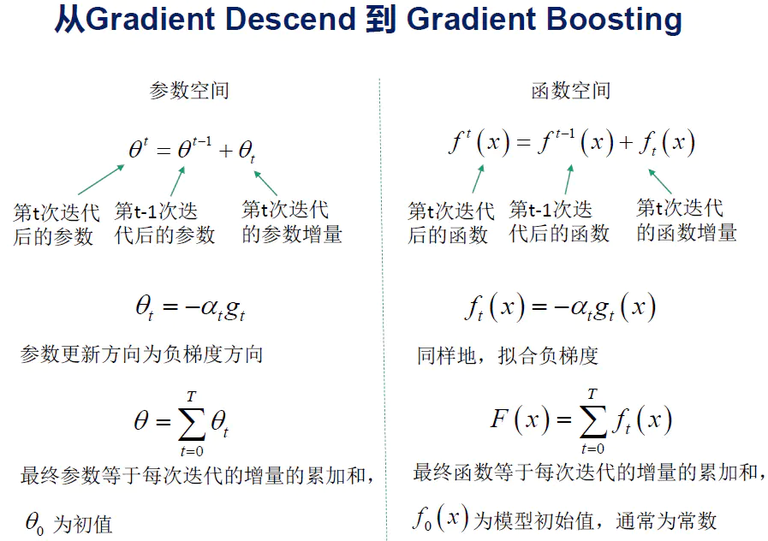
下面对目标函数进行泰勒公式二级展开、化简:
如果确定了树的结构,为了使目标函数最小,可以令其导数为0,解得每个叶节点的最优预测分数为:
代入目标函数,解得最小损失为:
3. 节点切分算法
注: 近似算法中使用到了分位数,关于分位数的选取,论文提出了一种算法Weighted Quantile Sketch 。XGBoost不是按照样本个数进行分位,而是以二阶导数为权重
在实际工作中,大多数输入是稀疏的。造成稀疏的原因有很多种,比如:缺失值、one-hot编码等。因此,论文提出为树中的节点设置一个默认方向来应对稀疏输入。论文实验表明稀疏感知算法 要比传统方法快50倍,算法如下:
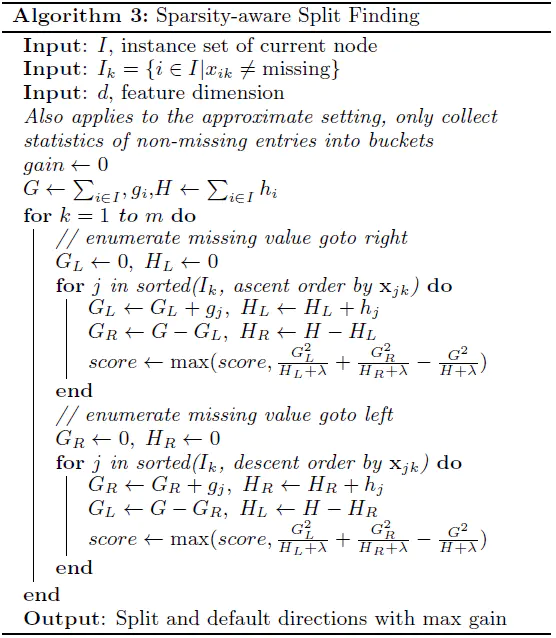
数据挖掘流程
1.数据清洗
数据集
- 这里涉及到的数据集是京东最新的数据集:
- JData_User.csv 用户数据集 105,321个用户
- JData_Comment.csv 商品评论 558,552条记录
- JData_Product.csv 预测商品集合 24,187条记录
- JData_Action_201602.csv 2月份行为交互记录 11,485,424条记录
- JData_Action_201603.csv 3月份行为交互记录 25,916,378条记录
- JData_Action_201604.csv 4月份行为交互记录 13,199,934条记录
为了能够进行上述清洗,在此首先构造了简单的用户(user)行为特征和商品(item)行为特征,对应于两张表user_table和item_table
user_table¶
- user_table特征包括:
- user_id(用户id),age(年龄),sex(性别),
- user_lv_cd(用户级别),browse_num(浏览数),
- addcart_num(加购数),delcart_num(删购数),
- buy_num(购买数),favor_num(收藏数),
- click_num(点击数),buy_addcart_ratio(购买加购转化率),
- buy_browse_ratio(购买浏览转化率),
- buy_click_ratio(购买点击转化率),
- buy_favor_ratio(购买收藏转化率)
user_table前面5条数据如下表所示:
user_id | age | sex | user_lv_cd | browse_num | addcart_num | delcart_num | buy_num | favor_num | click_num | buy_addcart_ratio | buy_browse_ratio | buy_click_ratio | buy_favor_ratio | |
---|---|---|---|---|---|---|---|---|---|---|---|---|---|---|
0 | 200001 | 6.0 | 2.0 | 5 | 212.0 | 22.0 | 13.0 | 1.0 | 0.0 | 414.0 | 0.045455 | 0.004717 | 0.002415 | 1.0 |
1 | 200002 | -1.0 | 0.0 | 1 | 238.0 | 1.0 | 0.0 | 0.0 | 0.0 | 484.0 | 0.000000 | 0.000000 | 0.000000 | NaN |
2 | 200003 | 4.0 | 1.0 | 4 | 221.0 | 4.0 | 1.0 | 0.0 | 1.0 | 420.0 | 0.000000 | 0.000000 | 0.000000 | 0.0 |
3 | 200004 | -1.0 | 2.0 | 1 | 52.0 | 0.0 | 0.0 | 0.0 | 0.0 | 61.0 | NaN | 0.000000 | 0.000000 | NaN |
4 | 200005 | 2.0 | 0.0 | 4 | 106.0 | 2.0 | 3.0 | 1.0 | 2.0 | 161.0 | 0.500000 | 0.009434 | 0.006211 | 0.5 |
item_table特征包括:
- sku_id(商品id),attr1,attr2,
- attr3,cate,brand,browse_num,
- addcart_num,delcart_num,
- buy_num,favor_num,click_num,
- buy_addcart_ratio,buy_browse_ratio,
- buy_click_ratio,buy_favor_ratio,
- comment_num(评论数),
- has_bad_comment(是否有差评),
- bad_comment_rate(差评率)
item_table前面5条数据如下表所示:
sku_id | a1 | a2 | a3 | cate | brand | browse_num | addcart_num | delcart_num | buy_num | favor_num | click_num | buy_addcart_ratio | buy_browse_ratio | buy_click_ratio | buy_favor_ratio | comment_num | has_bad_comment | bad_comment_rate | |
---|---|---|---|---|---|---|---|---|---|---|---|---|---|---|---|---|---|---|---|
0 | 10 | 3 | 1 | 1 | 8 | 489 | NaN | NaN | NaN | NaN | NaN | NaN | NaN | NaN | NaN | NaN | NaN | NaN | NaN |
1 | 100002 | 3 | 2 | 2 | 8 | 489 | NaN | NaN | NaN | NaN | NaN | NaN | NaN | NaN | NaN | NaN | NaN | NaN | NaN |
2 | 100003 | 1 | -1 | -1 | 8 | 30 | NaN | NaN | NaN | NaN | NaN | NaN | NaN | NaN | NaN | NaN | NaN | NaN | NaN |
3 | 100006 | 1 | 2 | 1 | 8 | 545 | NaN | NaN | NaN | NaN | NaN | NaN | NaN | NaN | NaN | NaN | NaN | NaN | NaN |
4 | 10001 | -1 | 1 | 2 | 8 | 244 | NaN | NaN | NaN | NaN | NaN | NaN | NaN | NaN | NaN | NaN | NaN | NaN | NaN |
用户数据清洗
统计用户信息
user_id | age | sex | user_lv_cd | browse_num | addcart_num | delcart_num | buy_num | favor_num | click_num | buy_addcart_ratio | buy_browse_ratio | buy_click_ratio | buy_favor_ratio | |
---|---|---|---|---|---|---|---|---|---|---|---|---|---|---|
count | 105,321.000 | 105,318.000 | 105,318.000 | 105,321.000 | 105,180.000 | 105,180.000 | 105,180.000 | 105,180.000 | 105,180.000 | 105,180.000 | 72,129.000 | 105,172.000 | 103,197.000 | 45,986.000 |
mean | 252,661.000 | 2.773 | 1.113 | 3.850 | 180.466 | 5.471 | 2.434 | 0.459 | 1.045 | 291.222 | 0.147 | 0.005 | 0.009 | 0.552 |
std | 30,403.698 | 1.672 | 0.956 | 1.072 | 273.437 | 10.618 | 5.600 | 1.048 | 3.442 | 460.031 | 0.270 | 0.022 | 0.074 | 0.473 |
min | 200,001.000 | -1.000 | 0.000 | 1.000 | 0.000 | 0.000 | 0.000 | 0.000 | 0.000 | 0.000 | 0.000 | 0.000 | 0.000 | 0.000 |
25% | 226,331.000 | 3.000 | 0.000 | 3.000 | 40.000 | 0.000 | 0.000 | 0.000 | 0.000 | 59.000 | 0.000 | 0.000 | 0.000 | 0.000 |
50% | 252,661.000 | 3.000 | 2.000 | 4.000 | 94.000 | 2.000 | 0.000 | 0.000 | 0.000 | 148.000 | 0.000 | 0.000 | 0.000 | 1.000 |
75% | 278,991.000 | 4.000 | 2.000 | 5.000 | 212.000 | 6.000 | 3.000 | 1.000 | 0.000 | 342.000 | 0.167 | 0.002 | 0.001 | 1.000 |
max | 305,321.000 | 6.000 | 2.000 | 5.000 | 7,605.000 | 369.000 | 231.000 | 50.000 | 99.000 | 15,302.000 | 1.000 | 1.000 | 1.000 | 1.000 |
由上述统计信息发现: 第一行中根据User_id统计发现有105321个用户,发现有3个用户没有age,sex字段,而且根据浏览、加购、删购、购买等记录却只有105180条记录,说明存在用户无任何交互记录,因此可以删除上述用户。
删除没有age,sex字段的用户,删除无交互记录的用户,删除爬虫及惰性用户,删除无购买记录的用户,统计用户信息如下:
user_id | age | sex | user_lv_cd | browse_num | addcart_num | delcart_num | buy_num | favor_num | click_num | buy_addcart_ratio | buy_browse_ratio | buy_click_ratio | buy_favor_ratio | |
---|---|---|---|---|---|---|---|---|---|---|---|---|---|---|
count | 29,070.000 | 29,070.000 | 29,070.000 | 29,070.000 | 29,070.000 | 29,070.000 | 29,070.000 | 29,070.000 | 29,070.000 | 29,070.000 | 29,070.000 | 29,070.000 | 29,070.000 | 29,070.000 |
mean | 250,767.099 | 2.910 | 1.028 | 4.268 | 280.260 | 10.145 | 4.457 | 1.644 | 1.589 | 447.113 | 0.364 | 0.019 | 0.031 | 0.866 |
std | 29,998.870 | 1.492 | 0.959 | 0.809 | 325.129 | 13.443 | 6.998 | 1.420 | 4.294 | 530.994 | 0.320 | 0.038 | 0.137 | 0.282 |
min | 200,001.000 | -1.000 | 0.000 | 2.000 | 1.000 | 0.000 | 0.000 | 1.000 | 0.000 | 0.000 | 0.004 | 0.001 | 0.001 | 0.018 |
25% | 225,036.000 | 3.000 | 0.000 | 4.000 | 75.000 | 3.000 | 0.000 | 1.000 | 0.000 | 114.000 | 0.125 | 0.004 | 0.002 | 1.000 |
50% | 249,200.500 | 3.000 | 1.000 | 4.000 | 174.000 | 6.000 | 2.000 | 1.000 | 0.000 | 275.000 | 0.250 | 0.008 | 0.005 | 1.000 |
75% | 276,284.000 | 4.000 | 2.000 | 5.000 | 366.000 | 13.000 | 6.000 | 2.000 | 1.000 | 585.000 | 0.500 | 0.018 | 0.012 | 1.000 |
max | 305,318.000 | 6.000 | 2.000 | 5.000 | 5,007.000 | 288.000 | 158.000 | 50.000 | 69.000 | 8,156.000 | 1.000 | 1.000 | 1.000 | 1.000 |
最后这29070个用户为最终预测用户数据集
2.数据探索
周一到周日各天购买情况
一个月中各天购买量
2月
3月
4月
周一到周日商品类别销售情况
每月各类商品销售情况(只关注商品8)
3.特征工程
评论特征:
- 分时间段,
- 对评论数进行独热编码
sku_id | has_bad_comment | bad_comment_rate | comment_num_0 | comment_num_1 | comment_num_2 | comment_num_3 | comment_num_4 | |
---|---|---|---|---|---|---|---|---|
0 | 1000 | 1 | 0.0417 | 0 | 0 | 0 | 1 | 0 |
1 | 10000 | 0 | 0.0000 | 0 | 0 | 1 | 0 | 0 |
2 | 100011 | 1 | 0.0376 | 0 | 0 | 0 | 0 | 1 |
3 | 100018 | 0 | 0.0000 | 0 | 0 | 0 | 1 | 0 |
4 | 100020 | 0 | 0.0000 | 0 | 0 | 0 | 1 | 0 |
行为特征:
- 分时间段
- 对行为类别进行独热编码
- 分别按照用户-类别行为分组和用户-类别-商品行为分组统计,然后计算
- 用户对同类别下其他商品的行为计数
- 不同时间累积的行为计数(3,5,7,10,15,21,30
user_id | sku_id | cate | type | action_before_3_1.0_x | action_before_3_2.0_x | action_before_3_3.0_x | action_before_3_4.0_x | action_before_3_5.0_x | action_before_3_6.0_x | action_before_3_1.0_y | action_before_3_2.0_y | action_before_3_3.0_y | action_before_3_4.0_y | action_before_3_5.0_y | action_before_3_6.0_y | action_before_3_1_y | |
---|---|---|---|---|---|---|---|---|---|---|---|---|---|---|---|---|---|
0 | 200002.0 | 7199.0 | 4.0 | 6.0 | 6.0 | 0.0 | 0.0 | 0.0 | 0.0 | 0.0 | 48.0 | 0.0 | 0.0 | 0.0 | 0.0 | 60.0 | 42.0 |
1 | 200002.0 | 24369.0 | 7.0 | 66.0 | 12.0 | 0.0 | 0.0 | 0.0 | 0.0 | 9.0 | 12.0 | 0.0 | 0.0 | 0.0 | 0.0 | 9.0 | 0.0 |
2 | 200002.0 | 28973.0 | 4.0 | 120.0 | 12.0 | 0.0 | 0.0 | 0.0 | 0.0 | 18.0 | 48.0 | 0.0 | 0.0 | 0.0 | 0.0 | 60.0 | 36.0 |
3 | 200002.0 | 73364.0 | 4.0 | 72.0 | 18.0 | 0.0 | 0.0 | 0.0 | 0.0 | 9.0 | 48.0 | 0.0 | 0.0 | 0.0 | 0.0 | 60.0 | 30.0 |
4 | 200002.0 | 75588.0 | 5.0 | 60.0 | 6.0 | 0.0 | 0.0 | 0.0 | 0.0 | 9.0 | 12.0 | 0.0 | 0.0 | 0.0 | 0.0 | 18.0 | 6.0 |
累积用户特征:
- 分时间段
- 用户不同行为的
- 购买转化率
- 均值
user_id | user_action_3_1.0 | user_action_3_2.0 | user_action_3_3.0 | user_action_3_4.0 | user_action_3_5.0 | user_action_3_6.0 | user_action_3_1_ratio | user_action_3_1_mean | |
---|---|---|---|---|---|---|---|---|---|
0 | 200002.0 | 84.0 | 0.0 | 0.0 | 0.0 | 0.0 | 123.0 | -4.442651 | 28.0 |
1 | 200003.0 | 60.0 | 0.0 | 0.0 | 0.0 | 0.0 | 93.0 | -4.110874 | 20.0 |
2 | 200008.0 | 24.0 | 0.0 | 0.0 | 0.0 | 0.0 | 60.0 | -3.218876 | 8.0 |
3 | 200023.0 | 3.0 | 0.0 | 0.0 | 0.0 | 0.0 | 0.0 | -1.386294 | 1.0 |
4 | 200030.0 | 24.0 | 0.0 | 0.0 | 0.0 | 0.0 | 51.0 | -3.218876 | 8.0 |
用户近期行为特征:
- 在上面针对用户进行累积特征提取的基础上,分别提取用户近一个月、近三天的特征,然后提取一个月内用户除去最近三天的行为占据一个月的行为的比重
cate_4_type1 | cate_5_type1 | cate_6_type1 | cate_7_type1 | cate_8_type1 | cate_9_type1 | cate_10_type1 | cate_11_type1 | cate_4_type2 | cate_5_type2 | ... | cate_5_type6 | cate_6_type6 | cate_7_type6 | cate_8_type6 | cate_9_type6 | cate_10_type6 | cate_11_type6 | cate_action_sum | cate8_percentage | cate8_type1_percentage | |
---|---|---|---|---|---|---|---|---|---|---|---|---|---|---|---|---|---|---|---|---|---|
user_id | |||||||||||||||||||||
200002.0 | 48.0 | 12.0 | 0.0 | 12.0 | 12.0 | 0.0 | 0.0 | 0.0 | 0.0 | 0.0 | ... | 18.0 | 0.0 | 9.0 | 36.0 | 0.0 | 0.0 | 0.0 | 414.0 | 0.115942 | -1.877702 |
200003.0 | 24.0 | 0.0 | 0.0 | 0.0 | 36.0 | 0.0 | 0.0 | 0.0 | 0.0 | 0.0 | ... | 0.0 | 0.0 | 0.0 | 57.0 | 0.0 | 0.0 | 0.0 | 306.0 | 0.303922 | -0.499956 |
200008.0 | 0.0 | 0.0 | 0.0 | 24.0 | 0.0 | 0.0 | 0.0 | 0.0 | 0.0 | 0.0 | ... | 0.0 | 0.0 | 60.0 | 0.0 | 0.0 | 0.0 | 0.0 | 168.0 | 0.000000 | -3.218876 |
200023.0 | 0.0 | 0.0 | 0.0 | 0.0 | 3.0 | 0.0 | 0.0 | 0.0 | 0.0 | 0.0 | ... | 0.0 | 0.0 | 0.0 | 0.0 | 0.0 | 0.0 | 0.0 | 6.0 | 0.500000 | 0.000000 |
200030.0 | 24.0 | 0.0 | 0.0 | 0.0 | 0.0 | 0.0 | 0.0 | 0.0 | 0.0 | 0.0 | ... | 0.0 | 0.0 | 0.0 | 0.0 | 0.0 | 0.0 | 0.0 | 150.0 | 0.000000 | -3.218876 |
用户对同类别下各种商品的行为:
- 用户对各个类别的各项行为操作统计
- 用户对各个类别操作行为统计占对所有类别操作行为统计的比重
sku_id | product_action_1.0 | product_action_2.0 | product_action_3.0 | product_action_4.0 | product_action_5.0 | product_action_6.0 | product_action_1_ratio | |
---|---|---|---|---|---|---|---|---|
0 | 2.0 | 6.0 | 0.0 | 0.0 | 0.0 | 0.0 | 9.0 | -1.945910 |
1 | 37.0 | 6.0 | 0.0 | 0.0 | 0.0 | 0.0 | 9.0 | -1.945910 |
2 | 40.0 | 12.0 | 0.0 | 0.0 | 0.0 | 0.0 | 27.0 | -2.564949 |
3 | 50.0 | 24.0 | 0.0 | 6.0 | 0.0 | 0.0 | 42.0 | -3.218876 |
4 | 52.0 | 261.0 | 0.0 | 3.0 | 0.0 | 0.0 | 336.0 | -5.568345 |
4.xgboost模型
训练数据集部分数据如下:
user_id | sku_id | cate | action_before_3_1.0_x | action_before_3_2.0_x | action_before_3_3.0_x | action_before_3_4.0_x | action_before_3_5.0_x | action_before_3_6.0_x | action_before_3_1.0_y | action_before_3_2.0_y | action_before_3_3.0_y | action_before_3_4.0_y | action_before_3_5.0_y | action_before_3_6.0_y | action_before_3minus_mean_1 | action_before_3minus_mean_2 | action_before_3minus_mean_3 | action_before_3minus_mean_4 | action_before_3minus_mean_5 | action_before_3minus_mean_6 | action_before_5_1.0_x | action_before_5_2.0_x | action_before_5_3.0_x | action_before_5_4.0_x | action_before_5_5.0_x | action_before_5_6.0_x | action_before_5_1.0_y | action_before_5_2.0_y | action_before_5_3.0_y | action_before_5_4.0_y | action_before_5_5.0_y | action_before_5_6.0_y | action_before_5minus_mean_1 | action_before_5minus_mean_2 | action_before_5minus_mean_3 | action_before_5minus_mean_4 | action_before_5minus_mean_5 | action_before_5minus_mean_6 | action_before_7_1.0_x | action_before_7_2.0_x | action_before_7_3.0_x | action_before_7_4.0_x | action_before_7_5.0_x | action_before_7_6.0_x | action_before_7_1.0_y | action_before_7_2.0_y | action_before_7_3.0_y | action_before_7_4.0_y | action_before_7_5.0_y | action_before_7_6.0_y | action_before_7minus_mean_1 | action_before_7minus_mean_2 | action_before_7minus_mean_3 | action_before_7minus_mean_4 | action_before_7minus_mean_5 | action_before_7minus_mean_6 | action_before_10_1.0_x | action_before_10_2.0_x | action_before_10_3.0_x | action_before_10_4.0_x | action_before_10_5.0_x | action_before_10_6.0_x | action_before_10_1.0_y | action_before_10_2.0_y | action_before_10_3.0_y | action_before_10_4.0_y | action_before_10_5.0_y | action_before_10_6.0_y | action_before_10minus_mean_1 | action_before_10minus_mean_2 | action_before_10minus_mean_3 | action_before_10minus_mean_4 | action_before_10minus_mean_5 | action_before_10minus_mean_6 | action_before_15_1.0_x | action_before_15_2.0_x | action_before_15_3.0_x | action_before_15_4.0_x | action_before_15_5.0_x | action_before_15_6.0_x | action_before_15_1.0_y | action_before_15_2.0_y | action_before_15_3.0_y | action_before_15_4.0_y | action_before_15_5.0_y | action_before_15_6.0_y | action_before_15minus_mean_1 | action_before_15minus_mean_2 | action_before_15minus_mean_3 | action_before_15minus_mean_4 | action_before_15minus_mean_5 | action_before_15minus_mean_6 | action_before_21_1.0_x | action_before_21_2.0_x | action_before_21_3.0_x | action_before_21_4.0_x | action_before_21_5.0_x | action_before_21_6.0_x | action_before_21_1.0_y | action_before_21_2.0_y | action_before_21_3.0_y | action_before_21_4.0_y | action_before_21_5.0_y | action_before_21_6.0_y | action_before_21minus_mean_1 | action_before_21minus_mean_2 | action_before_21minus_mean_3 | action_before_21minus_mean_4 | action_before_21minus_mean_5 | action_before_21minus_mean_6 | action_before_30_1.0_x | action_before_30_2.0_x | action_before_30_3.0_x | action_before_30_4.0_x | action_before_30_5.0_x | action_before_30_6.0_x | action_before_30_1.0_y | action_before_30_2.0_y | action_before_30_3.0_y | action_before_30_4.0_y | action_before_30_5.0_y | action_before_30_6.0_y | action_before_30minus_mean_1 | action_before_30minus_mean_2 | action_before_30minus_mean_3 | action_before_30minus_mean_4 | action_before_30minus_mean_5 | action_before_30minus_mean_6 | age_0 | age_1 | age_2 | age_3 | age_4 | age_5 | age_6 | sex_0 | sex_1 | sex_2 | user_lv_cd_1 | user_lv_cd_2 | user_lv_cd_3 | user_lv_cd_4 | user_lv_cd_5 | user_action_3_1.0 | user_action_3_2.0 | user_action_3_3.0 | user_action_3_4.0 | user_action_3_5.0 | user_action_3_6.0 | user_action_3_1_ratio | user_action_3_2_ratio | user_action_3_3_ratio | user_action_3_5_ratio | user_action_3_6_ratio | user_action_3_1_mean | user_action_3_2_mean | user_action_3_3_mean | user_action_3_4_mean | user_action_3_5_mean | user_action_3_6_mean | user_action_30_1.0 | user_action_30_2.0 | user_action_30_3.0 | user_action_30_4.0 | user_action_30_5.0 | user_action_30_6.0 | user_action_30_1_ratio | user_action_30_2_ratio | user_action_30_3_ratio | user_action_30_5_ratio | user_action_30_6_ratio | user_action_30_1_mean | user_action_30_2_mean | user_action_30_3_mean | user_action_30_4_mean | user_action_30_5_mean | user_action_30_6_mean | recent_action1 | recent_action2 | recent_action3 | recent_action4 | recent_action5 | recent_action6 | cate8_percentage | cate4_percentage | cate5_percentage | cate6_percentage | cate7_percentage | cate9_percentage | cate10_percentage | cate11_percentage | cate8_type1_percentage | cate8_type2_percentage | cate8_type3_percentage | cate8_type4_percentage | cate8_type5_percentage | cate8_type6_percentage | brand | a1_-1 | a1_1 | a1_2 | a1_3 | a2_-1 | a2_1 | a2_2 | a3_-1 | a3_1 | a3_2 | product_action_1.0 | product_action_2.0 | product_action_3.0 | product_action_4.0 | product_action_5.0 | product_action_6.0 | product_action_1_ratio | product_action_2_ratio | product_action_3_ratio | product_action_5_ratio | product_action_6_ratio | product_action_1_mean | product_action_2_mean | product_action_3_mean | product_action_4_mean | product_action_5_mean | product_action_6_mean | cate_action_1.0 | cate_action_2.0 | cate_action_3.0 | cate_action_4.0 | cate_action_5.0 | cate_action_6.0 | cate_action_1_ratio | cate_action_2_ratio | cate_action_3_ratio | cate_action_5_ratio | cate_action_6_ratio | cate_action_1_mean | cate_action_2_mean | cate_action_3_mean | cate_action_4_mean | cate_action_5_mean | cate_action_6_mean | has_bad_comment | bad_comment_rate | comment_num_0 | comment_num_1 | comment_num_2 | comment_num_3 | comment_num_4 | label |
202633 | 12564 | 8 | 3 | 0 | 0 | 0 | 0 | 6 | 3 | 0 | 0 | 0 | 0 | 3 | 2 | 0 | 0 | 0 | 0 | 4 | 3 | 0 | 0 | 0 | 0 | 6 | 3 | 0 | 0 | 0 | 0 | 3 | 2.4 | 0 | 0 | 0 | 0 | 4.8 | 3 | 0 | 0 | 0 | 0 | 6 | 3 | 0 | 0 | 0 | 0 | 3 | 2.571428571 | 0 | 0 | 0 | 0 | 5.142857143 | 3 | 0 | 0 | 0 | 0 | 6 | 3 | 0 | 0 | 0 | 0 | 3 | 2.7 | 0 | 0 | 0 | 0 | 5.4 | 3 | 0 | 0 | 0 | 0 | 6 | 3 | 0 | 0 | 0 | 0 | 3 | 2.8 | 0 | 0 | 0 | 0 | 5.6 | 3 | 0 | 0 | 0 | 0 | 6 | 3 | 0 | 0 | 0 | 0 | 3 | 2.857142857 | 0 | 0 | 0 | 0 | 5.714285714 | 3 | 0 | 0 | 0 | 0 | 6 | 3 | 0 | 0 | 0 | 0 | 3 | 2.9 | 0 | 0 | 0 | 0 | 5.8 | 0 | 0 | 0 | 1 | 0 | 0 | 0 | 1 | 0 | 0 | 0 | 0 | 0 | 0 | 1 | 6 | 0 | 0 | 0 | 0 | 9 | -1.945910149 | 0 | 0 | 0 | -2.302585093 | 2 | 0 | 0 | 0 | 0 | 3 | 6 | 0 | 0 | 0 | 0 | 9 | -1.945910149 | 0 | 0 | 0 | -2.302585093 | 0.2 | 0 | 0 | 0 | 0 | 0.3 | -1.945910149 | 0 | 0 | 0 | 0 | -2.302585093 | 1 | 0 | 0 | 0 | 0 | 0 | 0 | 0 | 0 | 0 | 0 | 0 | 0 | 0 | 214 | 0 | 0 | 0 | 1 | 0 | 1 | 0 | 0 | 0 | 1 | 5763 | 249 | 66 | 6 | 45 | 9870 | -6.713476808 | -3.575550769 | -2.25878247 | -1.882731247 | -7.251446295 | 192.1 | 8.3 | 2.2 | 0.2 | 1.5 | 329 | 295572 | 10701 | 3873 | 756 | 1878 | 465084 | -5.967307871 | -2.648822665 | -1.632679591 | -0.909131746 | -6.42061221 | 9852.4 | 356.7 | 129.1 | 25.2 | 62.6 | 15502.8 | 1 | 0.026 | 0 | 0 | 0 | 0 | 1 | 1 |
218498 | 149854 | 8 | 12 | 0 | 0 | 0 | 0 | 12 | 6 | 0 | 0 | 0 | 0 | 12 | 8 | 0 | 0 | 0 | 0 | 8 | 12 | 0 | 0 | 0 | 0 | 12 | 6 | 0 | 0 | 0 | 0 | 12 | 9.6 | 0 | 0 | 0 | 0 | 9.6 | 12 | 0 | 0 | 0 | 0 | 12 | 6 | 0 | 0 | 0 | 0 | 12 | 10.28571429 | 0 | 0 | 0 | 0 | 10.28571429 | 12 | 0 | 0 | 0 | 0 | 12 | 6 | 0 | 0 | 0 | 0 | 12 | 10.8 | 0 | 0 | 0 | 0 | 10.8 | 12 | 0 | 0 | 0 | 0 | 12 | 6 | 0 | 0 | 0 | 0 | 12 | 11.2 | 0 | 0 | 0 | 0 | 11.2 | 12 | 0 | 0 | 0 | 0 | 12 | 6 | 0 | 0 | 0 | 0 | 12 | 11.42857143 | 0 | 0 | 0 | 0 | 11.42857143 | 12 | 0 | 0 | 0 | 0 | 12 | 6 | 0 | 0 | 0 | 0 | 12 | 11.6 | 0 | 0 | 0 | 0 | 11.6 | 0 | 0 | 0 | 0 | 0 | 1 | 0 | 0 | 0 | 1 | 0 | 0 | 0 | 0 | 1 | 30 | 0 | 0 | 0 | 0 | 33 | -3.433987204 | 0 | 0 | 0 | -3.526360525 | 10 | 0 | 0 | 0 | 0 | 11 | 30 | 0 | 0 | 0 | 0 | 33 | -3.433987204 | 0 | 0 | 0 | -3.526360525 | 1 | 0 | 0 | 0 | 0 | 1.1 | -3.433987204 | 0 | 0 | 0 | 0 | -3.526360525 | 0.666666667 | 0.333333333 | 0 | 0 | 0 | 0 | 0 | 0 | -0.489548225 | 0 | 0 | 0 | 0 | -0.3074847 | 800 | 0 | 1 | 0 | 0 | 0 | 0 | 1 | 0 | 1 | 0 | 1110 | 45 | 3 | 12 | 6 | 1722 | -4.448066432 | -1.263692039 | 1.178654996 | 0.619039208 | -4.886872879 | 37 | 1.5 | 0.1 | 0.4 | 0.2 | 57.4 | 295572 | 10701 | 3873 | 756 | 1878 | 465084 | -5.967307871 | -2.648822665 | -1.632679591 | -0.909131746 | -6.42061221 | 9852.4 | 356.7 | 129.1 | 25.2 | 62.6 | 15502.8 | 1 | 0.0403 | 0 | 0 | 0 | 0 | 1 | 1 |
221842 | 75877 | 8 | 9 | 0 | 0 | 0 | 0 | 15 | 237 | 0 | 0 | 0 | 0 | 360 | 6 | 0 | 0 | 0 | 0 | 10 | 9 | 0 | 0 | 0 | 0 | 15 | 237 | 0 | 0 | 0 | 0 | 360 | 7.2 | 0 | 0 | 0 | 0 | 12 | 9 | 0 | 0 | 0 | 0 | 15 | 237 | 0 | 0 | 0 | 0 | 360 | 7.714285714 | 0 | 0 | 0 | 0 | 12.85714286 | 9 | 0 | 0 | 0 | 0 | 15 | 237 | 0 | 0 | 0 | 0 | 360 | 8.1 | 0 | 0 | 0 | 0 | 13.5 | 9 | 0 | 0 | 0 | 0 | 15 | 237 | 0 | 0 | 0 | 0 | 360 | 8.4 | 0 | 0 | 0 | 0 | 14 | 9 | 0 | 0 | 0 | 0 | 15 | 237 | 0 | 0 | 0 | 0 | 360 | 8.571428571 | 0 | 0 | 0 | 0 | 14.28571429 | 9 | 0 | 0 | 0 | 0 | 15 | 237 | 0 | 0 | 0 | 0 | 360 | 8.7 | 0 | 0 | 0 | 0 | 14.5 | 0 | 0 | 0 | 0 | 1 | 0 | 0 | 0 | 0 | 1 | 0 | 0 | 0 | 0 | 1 | 258 | 0 | 0 | 0 | 0 | 393 | -5.556828062 | 0 | 0 | 0 | -5.976350909 | 86 | 0 | 0 | 0 | 0 | 131 | 258 | 0 | 0 | 0 | 0 | 393 | -5.556828062 | 0 | 0 | 0 | -5.976350909 | 8.6 | 0 | 0 | 0 | 0 | 13.1 | -5.556828062 | 0 | 0 | 0 | 0 | -5.976350909 | 0.953917051 | 0 | 0.046082949 | 0 | 0 | 0 | 0 | 0 | -0.047439725 | 0 | 0 | 0 | 0 | -0.046761766 | 545 | 0 | 1 | 0 | 0 | 0 | 1 | 0 | 0 | 0 | 1 | 2253 | 96 | 36 | 6 | 9 | 4299 | -5.774551546 | -2.628800829 | -1.665007764 | -0.356674944 | -6.420460153 | 75.1 | 3.2 | 1.2 | 0.2 | 0.3 | 143.3 | 295572 | 10701 | 3873 | 756 | 1878 | 465084 | -5.967307871 | -2.648822665 | -1.632679591 | -0.909131746 | -6.42061221 | 9852.4 | 356.7 | 129.1 | 25.2 | 62.6 | 15502.8 | 1 | 0.0245 | 0 | 0 | 0 | 0 | 1 | 1 |
222886 | 154636 | 8 | 60 | 3 | 0 | 0 | 0 | 78 | 30 | 0 | 0 | 0 | 0 | 42 | 40 | 2 | 0 | 0 | 0 | 52 | 60 | 3 | 0 | 0 | 0 | 78 | 30 | 0 | 0 | 0 | 0 | 42 | 48 | 2.4 | 0 | 0 | 0 | 62.4 | 60 | 3 | 0 | 0 | 0 | 78 | 30 | 0 | 0 | 0 | 0 | 42 | 51.42857143 | 2.571428571 | 0 | 0 | 0 | 66.85714286 | 60 | 3 | 0 | 0 | 0 | 78 | 30 | 0 | 0 | 0 | 0 | 42 | 54 | 2.7 | 0 | 0 | 0 | 70.2 | 60 | 3 | 0 | 0 | 0 | 78 | 30 | 0 | 0 | 0 | 0 | 42 | 56 | 2.8 | 0 | 0 | 0 | 72.8 | 60 | 3 | 0 | 0 | 0 | 78 | 30 | 0 | 0 | 0 | 0 | 42 | 57.14285714 | 2.857142857 | 0 | 0 | 0 | 74.28571429 | 60 | 3 | 0 | 0 | 0 | 78 | 30 | 0 | 0 | 0 | 0 | 42 | 58 | 2.9 | 0 | 0 | 0 | 75.4 | 1 | 0 | 0 | 0 | 0 | 0 | 0 | 1 | 0 | 0 | 0 | 0 | 0 | 0 | 1 | 90 | 3 | 0 | 0 | 0 | 120 | -4.510859507 | -1.386294361 | 0 | 0 | -4.795790546 | 30 | 1 | 0 | 0 | 0 | 40 | 90 | 3 | 0 | 0 | 0 | 120 | -4.510859507 | -1.386294361 | 0 | 0 | -4.795790546 | 3 | 0.1 | 0 | 0 | 0 | 4 | -4.510859507 | -1.386294361 | 0 | 0 | 0 | -4.795790546 | 1 | 0 | 0 | 0 | 0 | 0 | 0 | 0 | 0 | 0 | 0 | 0 | 0 | 0 | 545 | 0 | 1 | 0 | 0 | 0 | 1 | 0 | 0 | 1 | 0 | 6255 | 258 | 96 | 27 | 33 | 9435 | -5.409091772 | -2.224623552 | -1.242506468 | -0.194156014 | -5.82008293 | 208.5 | 8.6 | 3.2 | 0.9 | 1.1 | 314.5 | 295572 | 10701 | 3873 | 756 | 1878 | 465084 | -5.967307871 | -2.648822665 | -1.632679591 | -0.909131746 | -6.42061221 | 9852.4 | 356.7 | 129.1 | 25.2 | 62.6 | 15502.8 | 1 | 0.0208 | 0 | 0 | 0 | 0 | 1 | 1 |
235240 | 38222 | 8 | 90 | 3 | 0 | 0 | 0 | 84 | 165 | 0 | 0 | 0 | 0 | 210 | 60 | 2 | 0 | 0 | 0 | 56 | 90 | 3 | 0 | 0 | 0 | 84 | 165 | 0 | 0 | 0 | 0 | 210 | 72 | 2.4 | 0 | 0 | 0 | 67.2 | 90 | 3 | 0 | 0 | 0 | 84 | 165 | 0 | 0 | 0 | 0 | 210 | 77.14285714 | 2.571428571 | 0 | 0 | 0 | 72 | 90 | 3 | 0 | 0 | 0 | 84 | 165 | 0 | 0 | 0 | 0 | 210 | 81 | 2.7 | 0 | 0 | 0 | 75.6 | 90 | 3 | 0 | 0 | 0 | 84 | 165 | 0 | 0 | 0 | 0 | 210 | 84 | 2.8 | 0 | 0 | 0 | 78.4 | 90 | 3 | 0 | 0 | 0 | 84 | 165 | 0 | 0 | 0 | 0 | 210 | 85.71428571 | 2.857142857 | 0 | 0 | 0 | 80 | 90 | 3 | 0 | 0 | 0 | 84 | 165 | 0 | 0 | 0 | 0 | 210 | 87 | 2.9 | 0 | 0 | 0 | 81.2 | 0 | 0 | 0 | 0 | 1 | 0 | 0 | 1 | 0 | 0 | 0 | 0 | 0 | 0 | 1 | 381 | 6 | 3 | 0 | 0 | 411 | -5.945420609 | -1.945910149 | -1.386294361 | 0 | -6.021023349 | 127 | 2 | 1 | 0 | 0 | 137 | 381 | 6 | 3 | 0 | 0 | 411 | -5.945420609 | -1.945910149 | -1.386294361 | 0 | -6.021023349 | 12.7 | 0.2 | 0.1 | 0 | 0 | 13.7 | -5.945420609 | -1.945910149 | -1.386294361 | 0 | 0 | -6.021023349 | 0.689138577 | 0.04494382 | 0 | 0 | 0.265917603 | 0 | 0 | 0 | -0.400243164 | -0.559615788 | -1.386294361 | 0 | 0 | -0.334047993 | 489 | 0 | 0 | 0 | 1 | 0 | 0 | 1 | 0 | 0 | 1 | 1167 | 45 | 27 | 6 | 6 | 1758 | -5.117138014 | -1.882731247 | -1.386294361 | 0 | -5.526590596 | 38.9 | 1.5 | 0.9 | 0.2 | 0.2 | 58.6 | 295572 | 10701 | 3873 | 756 | 1878 | 465084 | -5.967307871 | -2.648822665 | -1.632679591 | -0.909131746 | -6.42061221 | 9852.4 | 356.7 | 129.1 | 25.2 | 62.6 | 15502.8 | 1 | 0.0166 | 0 | 0 | 0 | 0 | 1 | 1 |
测试数据集部分数据如下:
user_id | sku_id | cate | action_before_3_1.0_x | action_before_3_2.0_x | action_before_3_3.0_x | action_before_3_4.0_x | action_before_3_5.0_x | action_before_3_6.0_x | action_before_3_1.0_y | action_before_3_2.0_y | action_before_3_3.0_y | action_before_3_4.0_y | action_before_3_5.0_y | action_before_3_6.0_y | action_before_3minus_mean_1 | action_before_3minus_mean_2 | action_before_3minus_mean_3 | action_before_3minus_mean_4 | action_before_3minus_mean_5 | action_before_3minus_mean_6 | action_before_5_1.0_x | action_before_5_2.0_x | action_before_5_3.0_x | action_before_5_4.0_x | action_before_5_5.0_x | action_before_5_6.0_x | action_before_5_1.0_y | action_before_5_2.0_y | action_before_5_3.0_y | action_before_5_4.0_y | action_before_5_5.0_y | action_before_5_6.0_y | action_before_5minus_mean_1 | action_before_5minus_mean_2 | action_before_5minus_mean_3 | action_before_5minus_mean_4 | action_before_5minus_mean_5 | action_before_5minus_mean_6 | action_before_7_1.0_x | action_before_7_2.0_x | action_before_7_3.0_x | action_before_7_4.0_x | action_before_7_5.0_x | action_before_7_6.0_x | action_before_7_1.0_y | action_before_7_2.0_y | action_before_7_3.0_y | action_before_7_4.0_y | action_before_7_5.0_y | action_before_7_6.0_y | action_before_7minus_mean_1 | action_before_7minus_mean_2 | action_before_7minus_mean_3 | action_before_7minus_mean_4 | action_before_7minus_mean_5 | action_before_7minus_mean_6 | action_before_10_1.0_x | action_before_10_2.0_x | action_before_10_3.0_x | action_before_10_4.0_x | action_before_10_5.0_x | action_before_10_6.0_x | action_before_10_1.0_y | action_before_10_2.0_y | action_before_10_3.0_y | action_before_10_4.0_y | action_before_10_5.0_y | action_before_10_6.0_y | action_before_10minus_mean_1 | action_before_10minus_mean_2 | action_before_10minus_mean_3 | action_before_10minus_mean_4 | action_before_10minus_mean_5 | action_before_10minus_mean_6 | action_before_15_1.0_x | action_before_15_2.0_x | action_before_15_3.0_x | action_before_15_4.0_x | action_before_15_5.0_x | action_before_15_6.0_x | action_before_15_1.0_y | action_before_15_2.0_y | action_before_15_3.0_y | action_before_15_4.0_y | action_before_15_5.0_y | action_before_15_6.0_y | action_before_15minus_mean_1 | action_before_15minus_mean_2 | action_before_15minus_mean_3 | action_before_15minus_mean_4 | action_before_15minus_mean_5 | action_before_15minus_mean_6 | action_before_21_1.0_x | action_before_21_2.0_x | action_before_21_3.0_x | action_before_21_4.0_x | action_before_21_5.0_x | action_before_21_6.0_x | action_before_21_1.0_y | action_before_21_2.0_y | action_before_21_3.0_y | action_before_21_4.0_y | action_before_21_5.0_y | action_before_21_6.0_y | action_before_21minus_mean_1 | action_before_21minus_mean_2 | action_before_21minus_mean_3 | action_before_21minus_mean_4 | action_before_21minus_mean_5 | action_before_21minus_mean_6 | action_before_30_1.0_x | action_before_30_2.0_x | action_before_30_3.0_x | action_before_30_4.0_x | action_before_30_5.0_x | action_before_30_6.0_x | action_before_30_1.0_y | action_before_30_2.0_y | action_before_30_3.0_y | action_before_30_4.0_y | action_before_30_5.0_y | action_before_30_6.0_y | action_before_30minus_mean_1 | action_before_30minus_mean_2 | action_before_30minus_mean_3 | action_before_30minus_mean_4 | action_before_30minus_mean_5 | action_before_30minus_mean_6 | age_0 | age_1 | age_2 | age_3 | age_4 | age_5 | age_6 | sex_0 | sex_1 | sex_2 | user_lv_cd_1 | user_lv_cd_2 | user_lv_cd_3 | user_lv_cd_4 | user_lv_cd_5 | user_action_3_1.0 | user_action_3_2.0 | user_action_3_3.0 | user_action_3_4.0 | user_action_3_5.0 | user_action_3_6.0 | user_action_3_1_ratio | user_action_3_2_ratio | user_action_3_3_ratio | user_action_3_5_ratio | user_action_3_6_ratio | user_action_3_1_mean | user_action_3_2_mean | user_action_3_3_mean | user_action_3_4_mean | user_action_3_5_mean | user_action_3_6_mean | user_action_30_1.0 | user_action_30_2.0 | user_action_30_3.0 | user_action_30_4.0 | user_action_30_5.0 | user_action_30_6.0 | user_action_30_1_ratio | user_action_30_2_ratio | user_action_30_3_ratio | user_action_30_5_ratio | user_action_30_6_ratio | user_action_30_1_mean | user_action_30_2_mean | user_action_30_3_mean | user_action_30_4_mean | user_action_30_5_mean | user_action_30_6_mean | recent_action1 | recent_action2 | recent_action3 | recent_action4 | recent_action5 | recent_action6 | cate8_percentage | cate4_percentage | cate5_percentage | cate6_percentage | cate7_percentage | cate9_percentage | cate10_percentage | cate11_percentage | cate8_type1_percentage | cate8_type2_percentage | cate8_type3_percentage | cate8_type4_percentage | cate8_type5_percentage | cate8_type6_percentage | brand | a1_-1 | a1_1 | a1_2 | a1_3 | a2_-1 | a2_1 | a2_2 | a3_-1 | a3_1 | a3_2 | product_action_1.0 | product_action_2.0 | product_action_3.0 | product_action_4.0 | product_action_5.0 | product_action_6.0 | product_action_1_ratio | product_action_2_ratio | product_action_3_ratio | product_action_5_ratio | product_action_6_ratio | product_action_1_mean | product_action_2_mean | product_action_3_mean | product_action_4_mean | product_action_5_mean | product_action_6_mean | cate_action_1.0 | cate_action_2.0 | cate_action_3.0 | cate_action_4.0 | cate_action_5.0 | cate_action_6.0 | cate_action_1_ratio | cate_action_2_ratio | cate_action_3_ratio | cate_action_5_ratio | cate_action_6_ratio | cate_action_1_mean | cate_action_2_mean | cate_action_3_mean | cate_action_4_mean | cate_action_5_mean | cate_action_6_mean | has_bad_comment | bad_comment_rate | comment_num_0 | comment_num_1 | comment_num_2 | comment_num_3 | comment_num_4 |
200005 | 67444 | 4 | 6 | 0 | 0 | 0 | 0 | 9 | 78 | 3 | 0 | 3 | 0 | 90 | 4 | 0 | 0 | 0 | 0 | 6 | 18 | 0 | 0 | 0 | 0 | 27 | 156 | 6 | 0 | 3 | 6 | 237 | 14.4 | 0 | 0 | 0 | 0 | 21.6 | 18 | 0 | 0 | 0 | 0 | 27 | 156 | 6 | 0 | 3 | 6 | 237 | 15.42857143 | 0 | 0 | 0 | 0 | 23.14285714 | 18 | 0 | 0 | 0 | 0 | 27 | 156 | 6 | 9 | 3 | 6 | 237 | 16.2 | 0 | 0 | 0 | 0 | 24.3 | 18 | 0 | 0 | 0 | 0 | 27 | 168 | 6 | 9 | 3 | 6 | 249 | 16.8 | 0 | 0 | 0 | 0 | 25.2 | 18 | 0 | 0 | 0 | 0 | 27 | 168 | 6 | 9 | 3 | 6 | 249 | 17.14285714 | 0 | 0 | 0 | 0 | 25.71428571 | 18 | 0 | 0 | 0 | 0 | 27 | 168 | 6 | 9 | 3 | 6 | 249 | 17.4 | 0 | 0 | 0 | 0 | 26.1 | 0 | 0 | 1 | 0 | 0 | 0 | 0 | 1 | 0 | 0 | 0 | 0 | 0 | 1 | 0 | 84 | 3 | 0 | 3 | 0 | 99 | -3.056356895 | 0 | 1.386294361 | 1.386294361 | -3.218875825 | 28 | 1 | 0 | 1 | 0 | 33 | 186 | 6 | 9 | 3 | 6 | 276 | -3.844814256 | -0.559615788 | -0.916290732 | -0.559615788 | -4.237723145 | 6.2 | 0.2 | 0.3 | 0.1 | 0.2 | 9.2 | -0.596379629 | -0.559615788 | 0 | -1.386294361 | 0 | -0.442233956 | 0 | 1 | 0 | 0 | 0 | 0 | 0 | 0 | -4.442651256 | -1.386294361 | 0 | -1.386294361 | 0 | -4.605170186 | 0 | 0 | 0 | 0 | 0 | 0 | 0 | 0 | 0 | 0 | 0 | 13350 | 261 | 99 | 6 | 66 | 19326 | -7.553436418 | -3.622434355 | -2.659260037 | -2.25878247 | -7.923348212 | 445 | 8.7 | 3.3 | 0.2 | 2.2 | 644.2 | 2928963 | 82674 | 40374 | 6606 | 15243 | 4342590 | -6.094274363 | -2.526787566 | -1.810081088 | -0.83605629 | -6.488096761 | 97632.1 | 2755.8 | 1345.8 | 220.2 | 508.1 | 144753 | 1 | 0.0821 | 0 | 0 | 0 | 0 | 1 |
200005 | 72967 | 4 | 78 | 3 | 0 | 3 | 0 | 90 | 6 | 0 | 0 | 0 | 0 | 9 | 52 | 2 | 0 | 2 | 0 | 60 | 114 | 6 | 0 | 3 | 6 | 159 | 60 | 0 | 0 | 0 | 0 | 105 | 91.2 | 4.8 | 0 | 2.4 | 4.8 | 127.2 | 114 | 6 | 0 | 3 | 6 | 159 | 60 | 0 | 0 | 0 | 0 | 105 | 97.71428571 | 5.142857143 | 0 | 2.571428571 | 5.142857143 | 136.2857143 | 114 | 6 | 0 | 3 | 6 | 159 | 60 | 0 | 9 | 0 | 0 | 105 | 102.6 | 5.4 | 0 | 2.7 | 5.4 | 143.1 | 114 | 6 | 0 | 3 | 6 | 159 | 72 | 0 | 9 | 0 | 0 | 117 | 106.4 | 5.6 | 0 | 2.8 | 5.6 | 148.4 | 114 | 6 | 0 | 3 | 6 | 159 | 72 | 0 | 9 | 0 | 0 | 117 | 108.5714286 | 5.714285714 | 0 | 2.857142857 | 5.714285714 | 151.4285714 | 114 | 6 | 0 | 3 | 6 | 159 | 72 | 0 | 9 | 0 | 0 | 117 | 110.2 | 5.8 | 0 | 2.9 | 5.8 | 153.7 | 0 | 0 | 1 | 0 | 0 | 0 | 0 | 1 | 0 | 0 | 0 | 0 | 0 | 1 | 0 | 84 | 3 | 0 | 3 | 0 | 99 | -3.056356895 | 0 | 1.386294361 | 1.386294361 | -3.218875825 | 28 | 1 | 0 | 1 | 0 | 33 | 186 | 6 | 9 | 3 | 6 | 276 | -3.844814256 | -0.559615788 | -0.916290732 | -0.559615788 | -4.237723145 | 6.2 | 0.2 | 0.3 | 0.1 | 0.2 | 9.2 | -0.596379629 | -0.559615788 | 0 | -1.386294361 | 0 | -0.442233956 | 0 | 1 | 0 | 0 | 0 | 0 | 0 | 0 | -4.442651256 | -1.386294361 | 0 | -1.386294361 | 0 | -4.605170186 | 0 | 0 | 0 | 0 | 0 | 0 | 0 | 0 | 0 | 0 | 0 | 16470 | 444 | 135 | 63 | 129 | 26052 | -5.550473454 | -1.939191199 | -0.753771802 | -0.708651367 | -6.00900512 | 549 | 14.8 | 4.5 | 2.1 | 4.3 | 868.4 | 2928963 | 82674 | 40374 | 6606 | 15243 | 4342590 | -6.094274363 | -2.526787566 | -1.810081088 | -0.83605629 | -6.488096761 | 97632.1 | 2755.8 | 1345.8 | 220.2 | 508.1 | 144753 | 1 | 0.0196 | 0 | 0 | 0 | 0 | 1 |
200007 | 26229 | 9 | 6 | 0 | 0 | 0 | 0 | 6 | 36 | 0 | 0 | 0 | 0 | 27 | 4 | 0 | 0 | 0 | 0 | 4 | 6 | 0 | 0 | 0 | 0 | 6 | 36 | 0 | 0 | 0 | 0 | 27 | 4.8 | 0 | 0 | 0 | 0 | 4.8 | 6 | 0 | 0 | 0 | 0 | 6 | 36 | 0 | 0 | 0 | 0 | 27 | 5.142857143 | 0 | 0 | 0 | 0 | 5.142857143 | 6 | 0 | 0 | 0 | 0 | 6 | 36 | 0 | 0 | 0 | 0 | 27 | 5.4 | 0 | 0 | 0 | 0 | 5.4 | 6 | 0 | 0 | 0 | 0 | 6 | 36 | 0 | 0 | 0 | 0 | 27 | 5.6 | 0 | 0 | 0 | 0 | 5.6 | 6 | 0 | 0 | 0 | 0 | 6 | 42 | 0 | 0 | 0 | 0 | 36 | 5.714285714 | 0 | 0 | 0 | 0 | 5.714285714 | 6 | 0 | 0 | 0 | 0 | 6 | 42 | 0 | 0 | 0 | 0 | 36 | 5.8 | 0 | 0 | 0 | 0 | 5.8 | 0 | 0 | 0 | 0 | 1 | 0 | 0 | 0 | 0 | 1 | 0 | 0 | 1 | 0 | 0 | 42 | 0 | 0 | 0 | 0 | 33 | -3.761200116 | 0 | 0 | 0 | -3.526360525 | 14 | 0 | 0 | 0 | 0 | 11 | 78 | 0 | 0 | 0 | 0 | 75 | -4.369447852 | 0 | 0 | 0 | -4.33073334 | 2.6 | 0 | 0 | 0 | 0 | 2.5 | -0.75852994 | 0 | 0 | 0 | 0 | -0.569533225 | 0 | 0 | 0 | 0 | 0 | 1 | 0 | 0 | -3.761200116 | 0 | 0 | 0 | 0 | -3.526360525 | 0 | 0 | 0 | 0 | 0 | 0 | 0 | 0 | 0 | 0 | 0 | 14031 | 312 | 174 | 27 | 81 | 19122 | -6.216891204 | -2.41399868 | -1.832581464 | -1.074514737 | -6.526442568 | 467.7 | 10.4 | 5.8 | 0.9 | 2.7 | 637.4 | 778755 | 24789 | 10650 | 1869 | 5103 | 1164393 | -6.031759344 | -2.584501915 | -1.739715354 | -1.004086115 | -6.434017628 | 25958.5 | 826.3 | 355 | 62.3 | 170.1 | 38813.1 | 1 | 0.0198 | 0 | 0 | 0 | 0 | 1 |
200007 | 63315 | 9 | 12 | 0 | 0 | 0 | 0 | 9 | 30 | 0 | 0 | 0 | 0 | 24 | 8 | 0 | 0 | 0 | 0 | 6 | 12 | 0 | 0 | 0 | 0 | 9 | 30 | 0 | 0 | 0 | 0 | 24 | 9.6 | 0 | 0 | 0 | 0 | 7.2 | 12 | 0 | 0 | 0 | 0 | 9 | 30 | 0 | 0 | 0 | 0 | 24 | 10.28571429 | 0 | 0 | 0 | 0 | 7.714285714 | 12 | 0 | 0 | 0 | 0 | 9 | 30 | 0 | 0 | 0 | 0 | 24 | 10.8 | 0 | 0 | 0 | 0 | 8.1 | 12 | 0 | 0 | 0 | 0 | 9 | 30 | 0 | 0 | 0 | 0 | 24 | 11.2 | 0 | 0 | 0 | 0 | 8.4 | 12 | 0 | 0 | 0 | 0 | 9 | 36 | 0 | 0 | 0 | 0 | 33 | 11.42857143 | 0 | 0 | 0 | 0 | 8.571428571 | 12 | 0 | 0 | 0 | 0 | 9 | 36 | 0 | 0 | 0 | 0 | 33 | 11.6 | 0 | 0 | 0 | 0 | 8.7 | 0 | 0 | 0 | 0 | 1 | 0 | 0 | 0 | 0 | 1 | 0 | 0 | 1 | 0 | 0 | 42 | 0 | 0 | 0 | 0 | 33 | -3.761200116 | 0 | 0 | 0 | -3.526360525 | 14 | 0 | 0 | 0 | 0 | 11 | 78 | 0 | 0 | 0 | 0 | 75 | -4.369447852 | 0 | 0 | 0 | -4.33073334 | 2.6 | 0 | 0 | 0 | 0 | 2.5 | -0.75852994 | 0 | 0 | 0 | 0 | -0.569533225 | 0 | 0 | 0 | 0 | 0 | 1 | 0 | 0 | -3.761200116 | 0 | 0 | 0 | 0 | -3.526360525 | 0 | 0 | 0 | 0 | 0 | 0 | 0 | 0 | 0 | 0 | 0 | 969 | 48 | 15 | 0 | 6 | 1602 | -6.877296071 | -3.891820298 | -2.772588722 | -1.945910149 | -7.379632153 | 32.3 | 1.6 | 0.5 | 0 | 0.2 | 53.4 | 778755 | 24789 | 10650 | 1869 | 5103 | 1164393 | -6.031759344 | -2.584501915 | -1.739715354 | -1.004086115 | -6.434017628 | 25958.5 | 826.3 | 355 | 62.3 | 170.1 | 38813.1 | 1 | 0.0476 | 0 | 0 | 0 | 0 | 1 |
模型训练信息如下:
Will train until eval-auc hasn't improved in 10 rounds. [1] train-auc:0.948637 eval-auc:0.942691 [2] train-auc:0.94976 eval-auc:0.942787 [3] train-auc:0.955299 eval-auc:0.950353 [4] train-auc:0.957374 eval-auc:0.951207 ...... [263] train-auc:0.992454 eval-auc:0.974031 [264] train-auc:0.992513 eval-auc:0.974034 [265] train-auc:0.992531 eval-auc:0.974071 Stopping. Best iteration: [255] train-auc:0.992115 eval-auc:0.974271
模型评估信息:
所有用户中预测购买商品的准确率为 0.556910569105691 所有用户中预测购买商品的召回率0.9513888888888888 F11=0.5755395683453236 F12=0.7413419913419912 score=0.6750210221433242